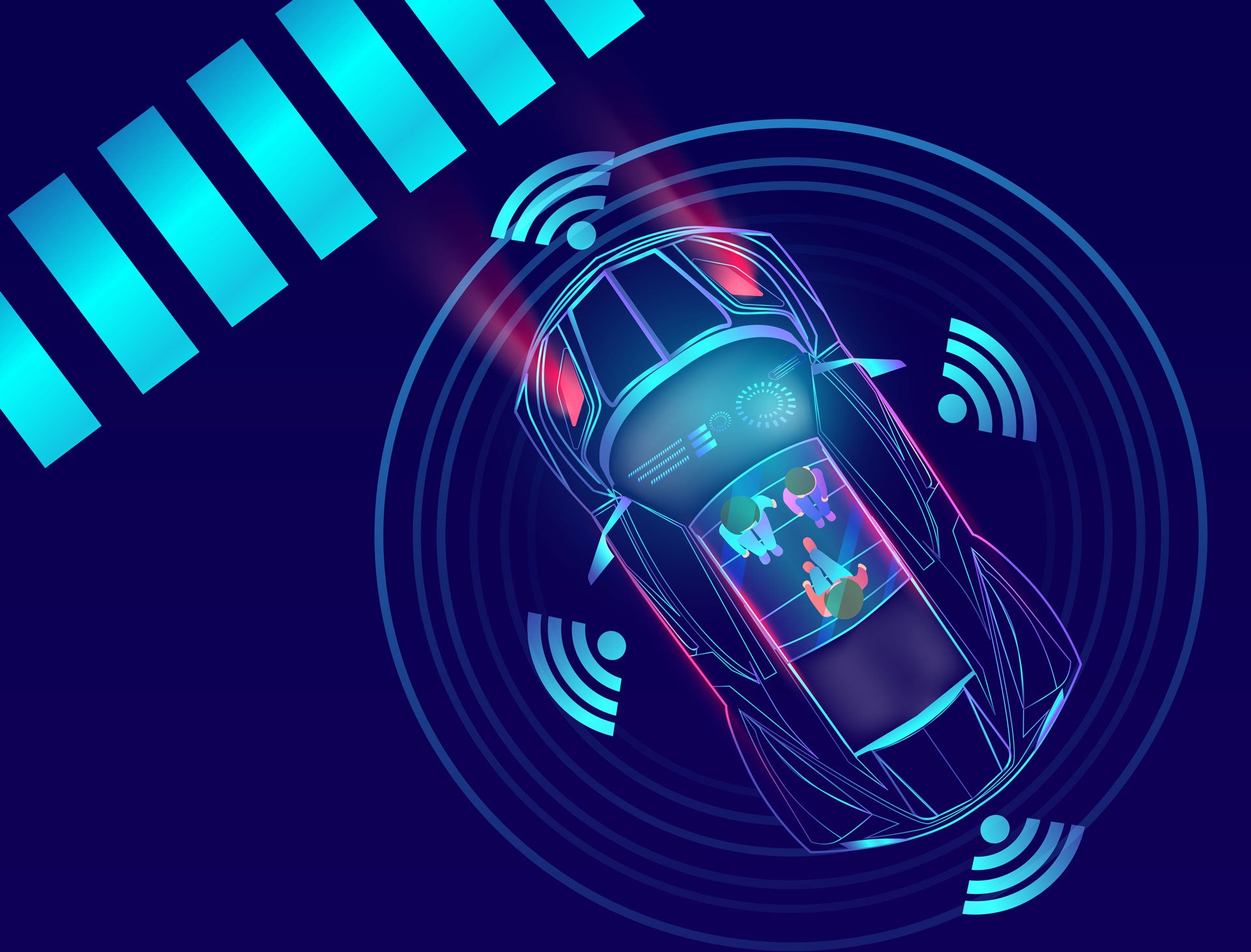
Autonomous vehicle (AV) pilots and small-scale roll outs have been brought into the mainstream in recent years by high profile brands such as Uber and Google’s Waymo. In 2018, the autonomous/driverless car market was valued at $5.68bn and is expected to project a CAGR of 31.50% during the forecast period 2019-2024.
Autonomous cars use technologies like RADAR, LIDAR, GPS and computer vision to sense their environment. Advanced control systems that are integrated into the car can interpret the sensory inputs to detect the signboards or to avoid the collision.
Although Level 4 and Level 5 autonomous cars are unlikely to reach mainstream acceptance, by 2030, there will be rapid growth for Level 2 and Level 3 autonomous cars, which have advanced driver assistance systems, like collision detection, lane departure warning, and adaptive cruise control.
However, in order to reach a stage where autonomous cars can gain mass market traction, the industry needs to meet the challenge of increasingly complex use cases and scenarios. Fully autonomous cars are not going to reach a wide customer base, unless, they are secure from cyber-attacks and near human like response in critical decision-making situations. If such concerns are addressed, by 2030 the autonomous car market is estimated to reach $60bn.
The technology within self-driving cars
The automotive industry is currently looking at the technology innovation needed to move from today’s level 2 or level 3 autonomous vehicle, to deployable safe and self-driving solutions. This technology must be able to tackle the key challenges that are currently preventing us from reaching and producing safe level 4 and level 5 autonomous vehicles.
The complexity of autonomous automotive systems is growing at an unprecedented rate, and computational processing must keep pace with this growth without compromising the current challenges of power consumption, thermal properties, size and cost, safety and security. To add to these challenges, there are still many debates about consumer and regulatory acceptance to address. Some of the challenges for large scale commercial deployment of autonomous vehicles include:
How well do you really know your competitors?
Access the most comprehensive Company Profiles on the market, powered by GlobalData. Save hours of research. Gain competitive edge.
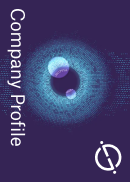
Thank you!
Your download email will arrive shortly
Not ready to buy yet? Download a free sample
We are confident about the unique quality of our Company Profiles. However, we want you to make the most beneficial decision for your business, so we offer a free sample that you can download by submitting the below form
By GlobalData- Cost price of autonomous vehicles
- Is autonomous vehicle deployment at level 3 a reality?
- Safety and software concerns
- Enhanced in-car passenger experiences
There are numerous challenges that will need to be overcome to get autonomous cars on the road. But progress is being made through an industry wide effort from automakers, engineers and problem solvers across multiple disciplines.
ADAS: The technology behind AVs
Advanced driver assistance systems (ADAS) are the first step toward a fully automated future. Features like emergency braking and adaptive cruise control are already helping drivers on the road and work to reduce the risk of error. But the performance of these systems isn’t always perfect. That’s why before hitting the road, advanced driver assistance systems cars have to go through different testing processes to prove their safety capabilities.
The common way to examine any car is to hold test drives. These can happen in different places such as highways, cities, or special test tracks. Test drives are useful for autonomous car testing because they can measure a vehicle’s performance in real-world situations.
However, unlike with traditional vehicles, testing autonomous vehicles differs from testing ordinary cars. Real-world testing simply cannot cover many of the unexpected scenarios that exist as potential variables. Because of this, evaluating a car’s on-road performance isn’t the best method for ADAS testing.
Moreover, real-world tests are expensive and time-consuming. Not to mention that test drives are dangerous for everyone, especially the driver. When it comes to autonomous, road testing alone does not ensure a vehicle’s safety. In fact, real-world test drives are the last step in the ADAS development cycle. So how do these vehicles get tested? The answer is in a lab.
The safe way of testing an autonomous car
To verify and test ADAS features, OEMs use virtual environment simulations, X-in-the-loop approaches, and augmentation of measured data. These testing methods lower risks and cut production costs in the earlier stages of SDLC.
Virtual environment simulation
Creating a virtual environment for ADAS testing means modelling a complete driving scenario using software. This includes the driver, sensors, traffic, and realistic vehicle dynamics. In contrast with real-world testing, virtual environment simulation is safe. It also allows the testing of self-driving cars in hundreds of thousands of potential scenarios. A virtual environment helps to validate many aspects of vehicles at a time, decreasing development costs where possible.
A by-product of this method means that virtual environments for ADAS testing helps to prototype and develop new system features. This can then help researchers create more reliable ADAS features and integrate different advanced driver assistance systems to develop better autonomous driving technology.

X-in-the-loop simulation methods
X-in-the-loop approaches usually combine both real-world and simulated elements for ADAS and autonomous car testing. Thanks to these methods, auto manufacturers can check the performance of specific car components early in development. Different tests like SIL, HIL, DIL, VEHIL & VIL method are normally used in for testing of autonomous vehicle systems.
Autonomous car testing ensures vehicle quality and ultimately helps to save lives. But since real-world testing of self-driving vehicles is too dangerous and expensive, OEMs need to test autonomous cars in labs and not on the road. Within labs, virtual environments, X-in-the-loop methods, and augmentation of measured data are safe ways of testing an autonomous car.
They help auto manufacturers create prototypes and find mistakes early in the development stage, saving both time and money. But most importantly, the testing of autonomous vehicle systems makes the road safer for everyone. To achieve accuracy in virtual simulation, testing companies need to invest in the following:
- Research and development of technology nuggets – algorithms, AUT, validation frameworks and frameworks for complying with AUTOSAR (the automotive industry’s architecture standard for electronic control units inside a vehicle).
- Partnerships with hardware manufacturers, sensor manufacturers, semiconductor vendors, and platform providers
- Adoption of new digital technologies such as AI and machine learning across the Verification and Validation phases.
Safer, faster testing of ADAS and autonomous systems
Some of the latest software is used to train, test and validate supervise autonomous driving models and ADAS systems. Simulated training data sets allow AI to experience life threatening situations without risk to real road users. Simulated testing allows users to push their AI to the limit, even in situations where the experiments are life threatening for passengers and other road users.
Autonomous vehicles will bring significant comfort benefits to passengers. However, safety cannot be compromised for alternative seating positions. Human modelling and simulation is currently the only technology that will allow assessment of the occupant’s protection for new car interior architectures with flexible seat arrangements.
The application of numerical simulations incorporating digital human models offers exciting opportunities in automotive development. Applying human models in comfort, ergonomics and safety allows the industry to overcome the limitations of using real humans or test dummies and enables greater optimisation of automotive designs. For instance, companies like DYNAmore, ESI Group, IAT, rFpro and Siemens offer different software for Human modelling and behaviour for simulation and virtual testing.
The virtual human body model is a Finite Element (FE) computer generated model of the human body designed to replicate its biomechanics and kinematics in a variety of scenarios. Elemance offers a variety of models including occupant, pedestrian, detailed and simplified versions. The creation of a Hybrid Twin, leveraging simulation, physics and data analytics, enables manufacturers to deliver smarter and connected products, to predict product performance and to anticipate maintenance needs.
Meanwhile, ESI delivers Virtual Prototyping as the foundation for the Hybrid Twin, synchronised through the Internet of Things (IoT) and Big Data to receive real-life product feedback to maximise the longevity of a product through intelligent predictive maintenance. Applying human models in comfort, ergonomics, occupant safety and other disciplines promises to overcome limitations imposed by using real humans or test dummies and enables optimisation of automotive designs.
The road ahead
The complete end-to-end physically modelled simulation can also be used for training AI, regression testing and identifying new failure modes. Full end-to-end testing also means users can involve their target customers – the passengers – in simulated testing. Involving passengers at the earliest possible stage helps ensure customer acceptance, and by extension protects investment in AI and autonomy.
A good driving first experience with an autonomous vehicle is critical, the automotive industry isn’t shying away from investing in the technologies and ecosystems that will get them there. Using virtual testing, automotive organisations will reduce costs, risk and time to market. Safety, reliability and robustness will be the prerequisite features for autonomous driving to achieve market success.
The role of modelling, simulation and testing is key to having safer and secure autonomous vehicles on the road. The vehicle of tomorrow will be an IOT software platform in motion, throughout its lifecycle and will remain in perpetual development.
Read more: “Maximising safety through innovation”: How the UK is tackling driverless car regulation