Hardly a day goes by without a discussion of artificial intelligence (AI) in telecoms and its potential benefits for customer experience (CX). AI can model the experience for individual users and services; more importantly, it can predict a problem that will soon affect CX and signal the network to fix it before users notice the problem.
To date, most attention on AI has focused on the algorithms and models themselves, including related governance issues and staffing requirements skills that CSPs need to acquire to support them. But as they gain more and more experience with AI, CSPs are confronting the fact that they also have to solve data and access challenges: they must process the data fast enough for it to be useful, and they must expand access to AI benefits to non-data scientists.
Five challenges
As the fuel for CX AI, data must be identified, ingested, normalized, processed, and passed to the target system. There are five major challenges:
• Identifying Data Sources Old and New. To be most effective, AI applications require silo walls to fall so they can process all types of data. CSPs are now gaining experience in combining data from traditional functional siloes: the network, customer care, billing and charging, and so on. To truly assess customer experience, they are now reaching beyond their traditional data sources to other customer touch points, among them social network data and the fintech data produced by increasingly sophisticated mobile money applications.
• Bringing the Data into the System. Once the data is identified, the system must ingest it. This is harder than it might seem: in the telecoms world especially, there are myriad data formats and interfaces associated with decades of legacy systems. Traditionally, these systems have not prioritized easy data extraction, so each has its own idiosyncratic interfaces and formats.
• Making the Data Usable. As a consequence, much of the data that enters the system is relatively dirty: records may be missing, corrupted, or they may not match the documented formats. CSPs produce huge amounts of structured and unstructured data, so any modern system must handle both. Some CSPs talk about “data lake houses,” a combination of data lakes and data warehouses, to describe these data stores. CSPs are also exploring techniques like auto-tagging to make the data more consumable by AI systems.
How well do you really know your competitors?
Access the most comprehensive Company Profiles on the market, powered by GlobalData. Save hours of research. Gain competitive edge.
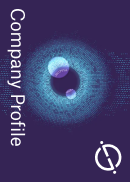
Thank you!
Your download email will arrive shortly
Not ready to buy yet? Download a free sample
We are confident about the unique quality of our Company Profiles. However, we want you to make the most beneficial decision for your business, so we offer a free sample that you can download by submitting the below form
By GlobalData• Processing Data in Real Time. AI becomes more valuable when its insight arrives at the time of need. Increasingly, CSPs have to process streaming and non-streaming data on the fly to support real-time applications, most notably service orchestration. This forces them to optimize the entire data chain, from initial data processing at the edge, to lightweight database architectures, to distributed architectures.
• Producing Output in Machine-usable Formats. Finally, the data must be output in a form usable by the target system. AI systems must therefore support not only dashboards and queries, but the variety of APIs and trigger formats used by orchestration, customer care, emergency, and other systems.
AI needs to avoid bottlenecks
This largely technical set of requirements is certainly enough to keep the data scientists and systems integrators busy, but there is another audience that must be addressed: business-side users – distinctly non-data scientists – who still require dashboards, reports, and queries to support their decisions. Any system that requires them to submit requests for information to the experts will create bottlenecks and decrease the CSP’s return on its substantial AI investment.
The AI system must therefore enable non-engineers to gain insight – via low- and no-code capabilities – from all the data ingestion and normalization work that the analytics team has done. These interfaces use various tools such as drag-and-drop functionality, configuration dropdowns, and SQL-like query languages. Special attention should be paid to enabling users to identify and select the most helpful data sources.
There are a few examples of products that do this well today, including Subex Hypersense, Oracle’s Cloud Scale Portfolio, and Huawei’s SmartCare. Executed properly, this approach can produce substantial business benefits: creating a single logical data store and pre-processing data in the source system, for example, can reduce data ingress/egress charges in a multiload architecture.
Just as important, an efficient, democratized, and consumable AI/data architecture will reap benefits in customer experience operations. Breaking down data siloes and enabling business-side queries will democratize access to the information, amortize the cost over more users and use cases, and enable data-driven decisions to catalyze organization-wide digital transformation.
Related Company Profiles
Oracle Corp