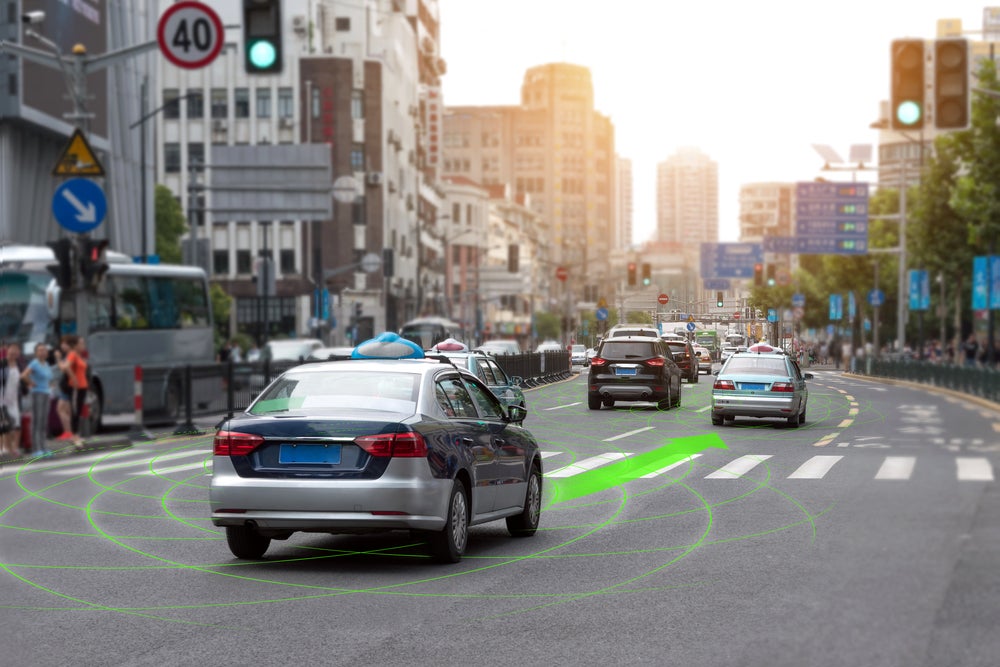
Self-driving cars are learning how to recognise and predict pedestrians’ movements with better accuracy, by focusing on people’s gait, body symmetry and foot placement.
The data for the cars was collected through cameras, LiDAR and GPS and let researchers from the University of Michigan catch video images of people in motion that could be recreated in a 3D computer simulation.
This was fed into a “biomechanically inspired recurrent neural network”, cataloguing human movement.
Self-driving cars used the catalogue to predict the position and locations of pedestrians at 50 yards from the vehicle.
“Prior work in this area has typically only looked at still images. It wasn’t really concerned with how people move in three dimensions,” said Ram Vasudevan, U-M assistant professor of Mechanical Engineering.
“But if these vehicles are going to operate and interact in the real world, we need to make sure our predictions of where a pedestrian is going doesn’t coincide with where the vehicle is going next.”
How well do you really know your competitors?
Access the most comprehensive Company Profiles on the market, powered by GlobalData. Save hours of research. Gain competitive edge.
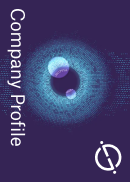
Thank you!
Your download email will arrive shortly
Not ready to buy yet? Download a free sample
We are confident about the unique quality of our Company Profiles. However, we want you to make the most beneficial decision for your business, so we offer a free sample that you can download by submitting the below form
By GlobalDataPeople are unlikely to fly, applied in machine learning to autonomise cars
The autonomous systems use machine learning on video clips that last a few seconds, studying the first half of the video to make predictions and verifying their accuracy with the second half.
The researchers also applied the physical constraints of the human body, such as the unlikelihood of taking off in flight and the fastest speeds possible by foot, to restrict the system’s predictions.
The researchers from U-M built the catalogue of human movement by recording at several Michigan intersections using a Level 4 autonomous car.
Before now, the autonomous tech would work with still imagery, such as using several million photos of stop signs to recognise an actual stops sign in real time and space.
“The median translation error of our prediction was approximately 10cm after one second and less than 80cm after six seconds. All other comparison methods were up to seven metres off,” said Matthew Johnson-Roberson, associate professor in U-M’s Department of Naval Architecture and Marine Engineering.
Ultimately, he said: “We’re better at figuring out where a person is going to be.”
People will programme an autonomous car to be more cooperative than them as drivers
Another research team that included the US Army’s Institute for Creative Technologies found that people would programme an autonomous car to behave in a more cooperative way than they would if they were driving the cars themselves.
The result contradicted researchers, who expected the involvement of AI in cars to make people more selfish.
The study involved over a thousand volunteers in computerised experiments involving a social dilemma, where individuals benefit from a selfish decision unless everyone in the group makes a selfish decision, to do with autonomous vehicles.
Dr Celso de Melo from the US Combat Capabilities Development Command’s Army Research Laboratory, who led the research, said: “Autonomous machines that act on people’s behalf — such as robots, drones and autonomous vehicles — are quickly becoming a reality and are expected to play an increasingly important role in the battlefield of the future.
“People are more likely to make unselfish decisions to favour collective interest when asked to programme autonomous machines ahead of time versus making the decision in real-time on a moment-to-moment basis.”