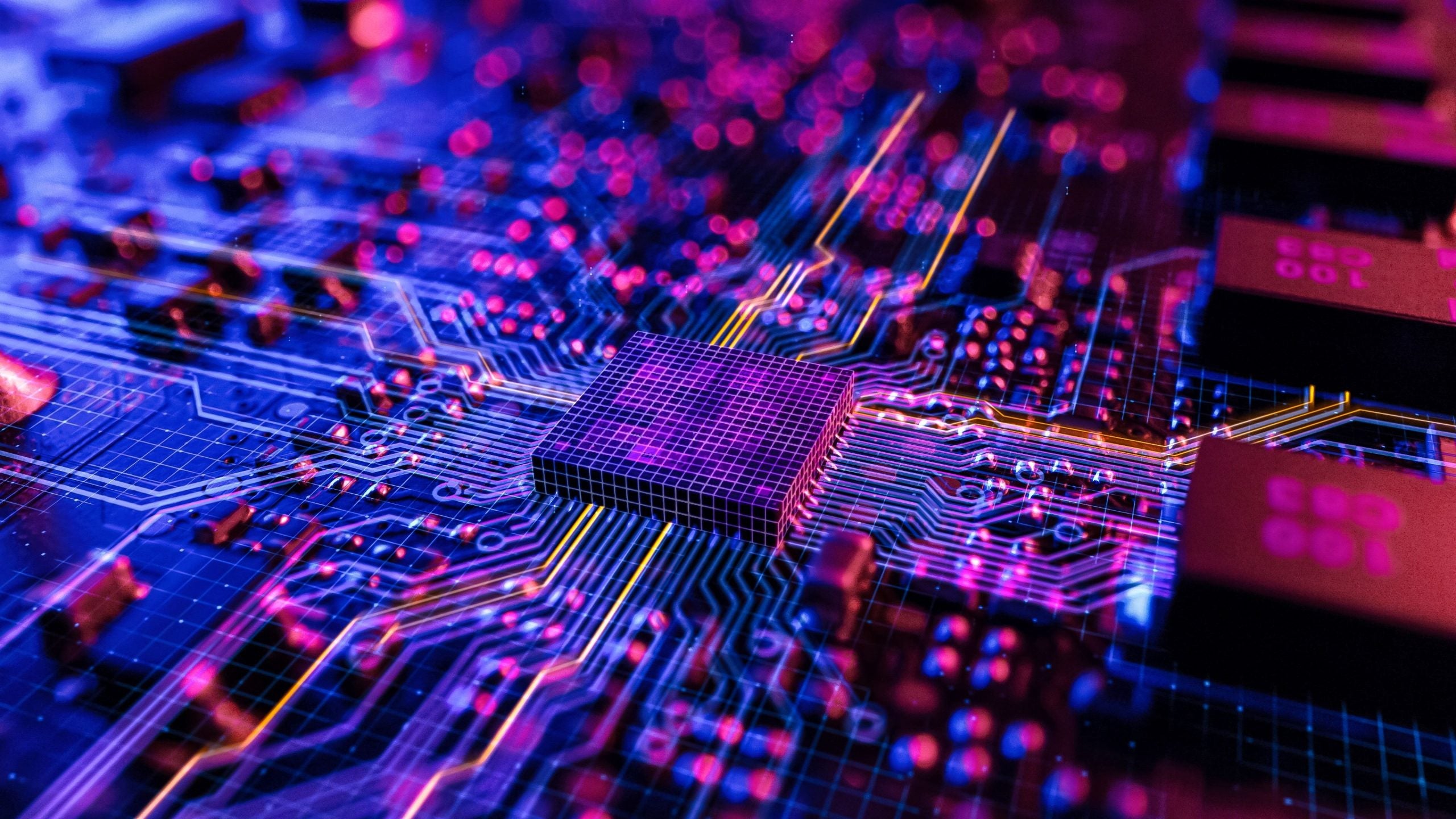
Researchers at the University of Oxford have successfully tackled a significant hurdle in the development of quantum devices by employing machine learning techniques.
A groundbreaking study introduces a method to close the ‘reality gap,’ the disparity between predicted and observed behaviour in quantum devices, opening new avenues for the advancement of quantum computing.
The findings, published in Physical Review X, offer promising insights for applications ranging from climate modelling to drug discovery.
In quantum computing, the scalability and integration of individual quantum devices, also known as qubits, are critical for enhanced functionality in various fields. However, the inherent variability among seemingly identical quantum units poses a major obstacle.
This variability is believed to stem from nanoscale imperfections in the materials used for quantum devices, and since these imperfections cannot be directly measured, predicting outcomes becomes challenging.
To address this issue, the research team adopted a “physics-informed” machine learning approach. Lead researcher Associate Professor Natalia Ares, from the University of Oxford’s Department of Engineering Science, explained the methodology using a playful analogy, likening it to improving predictions in a game of “crazy golf” through repeated shots, a simulator and machine learning.
The researchers gathered data by measuring the output current for various voltage settings across an individual quantum dot device. This data was then input into a simulation that calculated the differences between the measured and theoretical current, accounting for the absence of internal disorder.
By iterating this process for various voltage settings, the simulation successfully identified internal disorder configurations that explained the measurements. This approach combined mathematical and statistical techniques with deep learning.
Associate Professor Ares elaborated, “In the crazy golf analogy, it would be equivalent to placing a series of sensors along the tunnel so that we could take measurements of the ball’s speed at different points.
How well do you really know your competitors?
Access the most comprehensive Company Profiles on the market, powered by GlobalData. Save hours of research. Gain competitive edge.
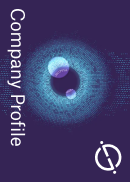
Thank you!
Your download email will arrive shortly
Not ready to buy yet? Download a free sample
We are confident about the unique quality of our Company Profiles. However, we want you to make the most beneficial decision for your business, so we offer a free sample that you can download by submitting the below form
By GlobalData“Although we still can’t see inside the tunnel, we can use the data to inform better predictions of how the ball will behave when we take the shot.”
Importantly, the developed model not only identified suitable internal disorder profiles but also accurately predicted voltage settings for specific device operating conditions.
The model’s ability to quantify variability between quantum devices could lead to more precise predictions of device performance and aid in the engineering of optimal materials for quantum devices. Furthermore, it may inform compensation strategies to mitigate the effects of material imperfections in quantum devices.
Co-author David Craig, a PhD student at the University of Oxford’s Department of Materials, drew parallels with the indirect observation of black holes, stating, “Similar to how we cannot observe black holes directly but infer their presence from their effect on surrounding matter, we have used simple measurements as a proxy for the internal variability of nanoscale quantum devices.
“Although the real device still has greater complexity than the model can capture, our study has demonstrated the utility of using physics-aware machine learning to narrow the reality gap.”
Research company GlobalData’s Thematic Intelligence: Quantum Computing report estimates that the quantum computing market in 2022 was valued between $500m and $1bn. The research company predicts this figure will rise to $10bn between 2026 and 2030, representing a compound annual growth rate of between 30% and 50%.