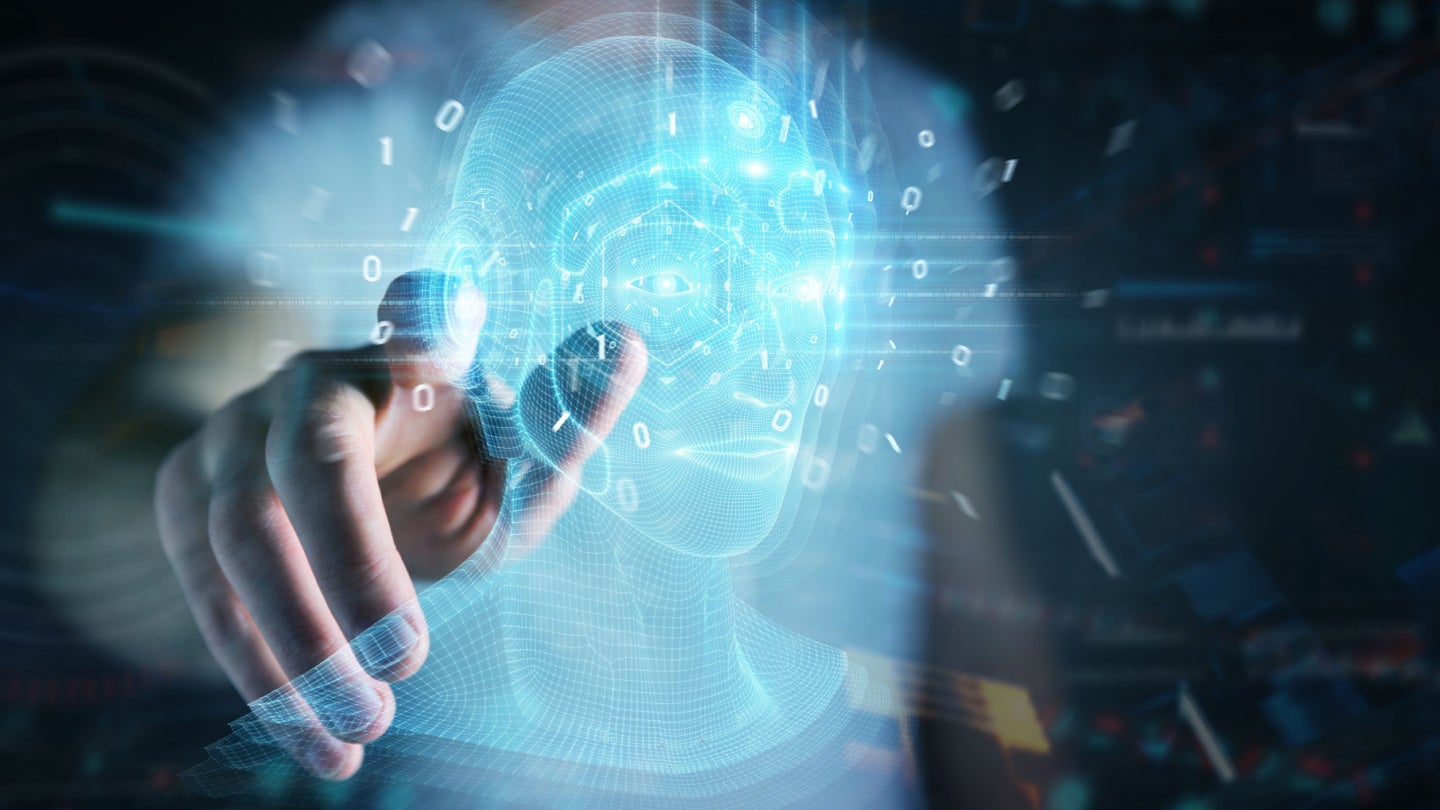
Although the use of artificial intelligence (AI) is accelerating in security, its increased use could actually invite AI cyber attacks (including adversarial attacks) in varied systems, devices and applications.
Recent advances around the improvement of algorithms (Google’s AlphaGo, OpenAI’s GPT-3), and increasing computing power have accelerated AI across a number of potential applications and use cases.
Use cases stem across Automotive (computer vision and conversational platforms), Consumer Electronics (implementing virtual assistants, authentication via facial recognition, i.e. Apple’s FaceID), and Ecommerce and Retail (voice-enabled shopping assistants, personalized shop). Accordingly, based on GlobalData forecasts, the total AI market is demonstrating strong growth (includes software, hardware, and services) and will be worth $383.3bn in 2030, having grown at a compound annual growth rate (CAGR) of 21.4% from $81.3bn in 2022. As a result many of these use cases will be across a number of consumer and business application settings.
What’s the role of AI/ML In Cybersecurity?
AI within cybersecurity is very much talked about in how AI can be utilized to increase cyber resiliency, simplify processes, and perform human functions. AI together with Automation and Analytics enables managed security providers to ingest data from multiple feeds and react more quickly to real threats, and apply automation to incidence response in a broader way.
AI in cybersecurity is also seen to solve the problem in the long run of resourcing, by in the short term providing a stop gap by streamlining human functions across Security Operations Centers (SOCs) – this could be through for example cybersecurity technology components covering Extended, Detection and Response (XDR) that detect sophisticated threats with AI; and Security Orchestration, Automation and Response (SOAR) platforms that utilize Machine Learning (ML) to provide incident handling guidance based on past actions and historical data.
Dangers of cyber attacks on AI
On the flip side, the increased use of AI in all applications (including cybersecurity) increases the chances of attacks on the actual AI/ML models in varied systems, devices and applications. In addition, adversarial attacks on AI could cause models to misinterpret information. There are many use cases that this could occur, and examples include iPhone’s “FaceID” access feature that makes use of neural networks to recognize faces – Here there is potential for attacks to happen through the AI models themselves and in bypassing the security layers.
How well do you really know your competitors?
Access the most comprehensive Company Profiles on the market, powered by GlobalData. Save hours of research. Gain competitive edge.
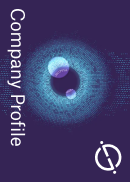
Thank you!
Your download email will arrive shortly
Not ready to buy yet? Download a free sample
We are confident about the unique quality of our Company Profiles. However, we want you to make the most beneficial decision for your business, so we offer a free sample that you can download by submitting the below form
By GlobalDataCybersecurity products where AI is implemented is also a target as AI in cybersecurity entails acquiring data sets over time which are vulnerable to attacks. Other examples include algorithm theft in autonomous vehicles, predictive maintenance algorithms in sectors like Oil &Gas and Utilities which could be subject to State Sponsored attacks, identification breaches in video surveillance, and medical misdiagnosis in Healthcare.
Countering attacks on AI in the future
The discussion of countering attacks on AI will gain momentum over the next two years as AI use cases increase. Regulations around AI security will also drive momentum and frameworks in place to address cyber attacks on AI.
As an example, current regulatory examples at a vertical level include The European Telecommunications Standards Institute (ETSI) Industry Specification Group for Telecoms that is focusing on utilizing AI to enhance security and securing AI attacks.
The Financial sector as a whole is in its infancy in terms of setting and implementing AI regulatory frameworks. Though, there have been developments in Europe for example, and the European Commission published a comprehensive set of proposals for the AI Act. However, the security component is limited.
The lack of guidance and regulation currently leaves a number of vertical sectors like Finance and Utilities vulnerable.
However, as more AI regulatory frameworks in the context of security are introduced, this could pave way to the rise of managed services specifically at addressing attacks on AI – service propositions could entail looking at risk management profiles and laying down security layers around vulnerability assessments, and better integration of MLOps and SIEM/SOAR environments.
Related Company Profiles
Apple Inc