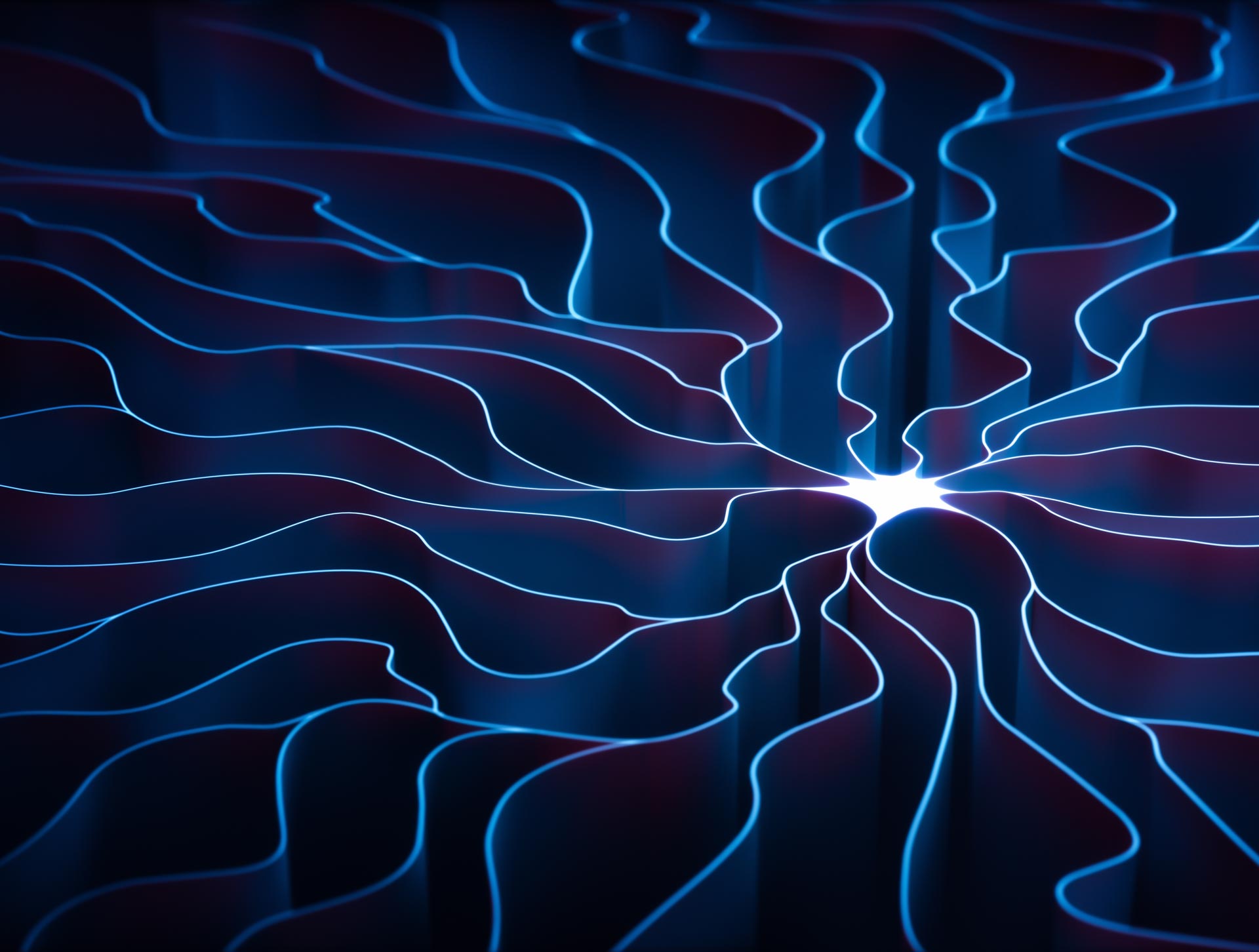
A current joke among data scientists is “If It’s built in Python it’s ML (machine learning), if it’s built in PowerPoint it’s AI.” Businesses have been talking a big game about industry advances through increasing automation, but in reality, AI is still far more notional than functional.
In fact recent articles have shown that one of the most widely hyped advantages of AI, increasing personalization and targeted advertising, barely performs better than the non-targeted kind and that in general marketing spend confuses correlation with causation: punters didn’t buy because we gave them a coupon or showed them a specific ad, they got a coupon or saw a specific ad because they were already likely to buy.
Beyond that, we’ve seen endless stories about AI discriminating against black patients when allocating health care resources, against the disabled in screening job applicants by video interview, and against women in determining credit limits even for married couples with identical credit scores and other financial information.
Nobody wants to be next in the headlines for this kind of discrimination, yet businesses and governments seem endlessly seduced by the promise of AI, despite extremely dubious rates of success and the immense cost of building and maintaining these systems in a market where data scientists command immense salaries.
AI in business: Where it is working
So where is it working? There are some real success stories in reducing time to completion and freeing up humans from repetitive tasks in various industries and predicting failure points in complex systems.
Examples include predictive maintenance for manufacturing and field equipment, predicting load balancing for complex systems like telecommunications infrastructure, improvements to transcription and translation software, and automating process flows like billing and payments, producing documents, and search and categorisation for massive amounts of paperwork.
How well do you really know your competitors?
Access the most comprehensive Company Profiles on the market, powered by GlobalData. Save hours of research. Gain competitive edge.
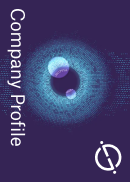
Thank you!
Your download email will arrive shortly
Not ready to buy yet? Download a free sample
We are confident about the unique quality of our Company Profiles. However, we want you to make the most beneficial decision for your business, so we offer a free sample that you can download by submitting the below form
By GlobalDataIn other words, it works best in environments where there is low risk of human rights violations because it’s working with abstract concepts like inventory or infrastructure rather than making decisions about people.
AI does have the potential to actually mitigate bias in many of the disastrous examples above, but at the moment it continues to replicate existing societal biases because that’s all we have to train it on. In a way, many of these scandals are simply pointing out in procedural terms the biases that we humans have introduced against one another.
It’s up to everyone involved in designing and building AI, from business leaders to software engineers, not to accept this state of affairs and allow it to be encoded into the fabric of our digital lives, to shrug our shoulders and say that the AI is only doing what we tell it to, but instead to push for a better world for all, online and off.
Read more: AI at work: No mass unemployment but change ahead, says Daniel Susskind