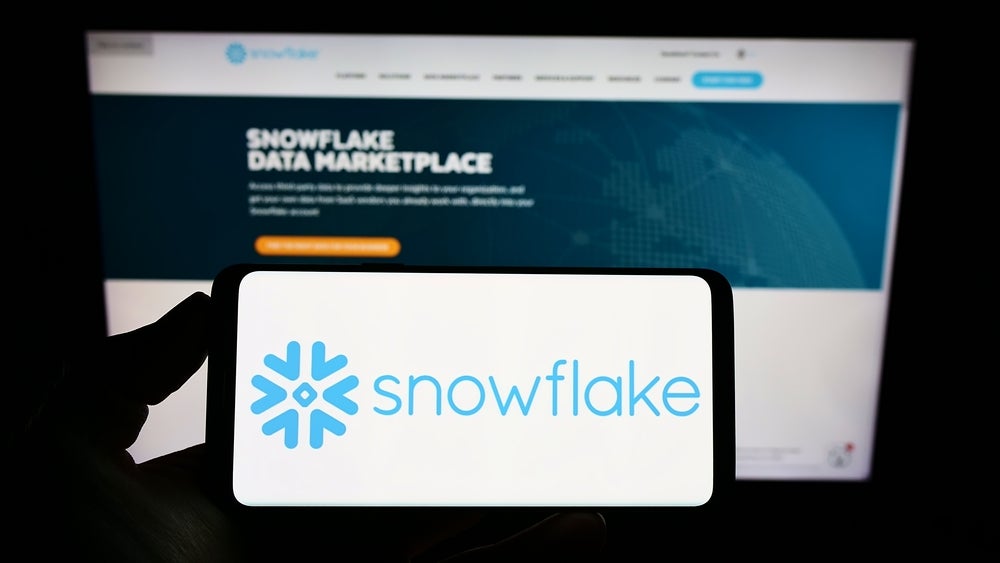
Before organisations get lost in the potential benefits of AI, they need to be acutely aware of the risks when deploying sensitive data into publicly hosted large language models (LLMs) in terms of security, privacy and governance. Businesses need to carefully consider these risks and implement an approach that keeps their data safe and ensures it adheres to current regulation.
The data governance rules around publicly available models are yet to be defined, but it is widely perceived that any data shared with LLMs through chat prompts is available to use. Understandably, enterprises have real concerns, about publicly hosted LLMs ‘learning’ from the prompts used by employees within a business, which might then resurface elsewhere. There are also worries that sharing sensitive data with LLMs could inadvertently expose a business to hackers.
For most enterprises, this means publicly hosted LLMs are not a viable option. This applies, even more, so for organisations in tightly regulated industries such as financial services or the public sector. So how can business leaders reap the value of this technology while better managing the risks?

Operate within a safe space
Most enterprises already have strict boundaries around their data when it comes to security and governance, and the same should apply when using LLMs. This means instead of sending data out to an LLM, they should bring the LLM to their data. By doing so, teams balance the need for innovation with the importance of keeping customer information and other sensitive data secure. It also allows data teams to customise the LLM to their needs while other employees interact with it, all within the organisation’s existing security parameter.
To create a strong AI strategy, businesses need a strong data strategy in the first instance and at its very foundation. That means implementing consistent, robust policies which allow the teams to access data safely within the security and governance posture, without data being siloed in different parts of the organisation. The end goal is to have actionable, trustworthy data that can be accessed easily to use with an LLM within a secure and governed environment.
Domain-specific LLMs
Publicly available LLMs such as ChatGPT pose additional challenges for enterprise users, as they are trained on the entire internet. LLMs trained on a wide variety of data are prone to ‘hallucinations’ and other inaccuracies, and are also alarmingly prone to bias or creating offensive answers. These foundational LLMs have also not been exposed to an organisation’s internal systems and data, and therefore can’t answer questions specific to a business.
How well do you really know your competitors?
Access the most comprehensive Company Profiles on the market, powered by GlobalData. Save hours of research. Gain competitive edge.
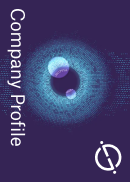
Thank you!
Your download email will arrive shortly
Not ready to buy yet? Download a free sample
We are confident about the unique quality of our Company Profiles. However, we want you to make the most beneficial decision for your business, so we offer a free sample that you can download by submitting the below form
By GlobalDataAs a solution, enterprises need to customise a model to make it smart about the specifics of the business and their customers’ needs. While hosted models such as ChatGPT have dominated headlines, there is a growing list of LLMs built for enterprises such as Llama v2 from Meta, StarCoder from Hugging Face and StableLM from StabilityAI. These can be customised to the specific needs of the business and used safely behind a firewall.
Optimising the business’ LLM to answer the right questions for the organisation has other benefits. Smaller models built for very specific use cases within an organisation require a smaller memory size and far less compute power than models built for more general-purpose uses. Models can be ‘fine-tuned’ to a particular business need, such as product demand, in much less time than it takes to train a general-purpose model, meaning the business can be more efficient and cost-effective.
Take advantage of multimodal AI
For any organisation looking to take full advantage of LLM technology, business leaders must ensure all of their useful data is available for the AI to work with. Around 80% of the world’s data is unstructured, and organisations are likely to have important data stored in formats besides text, such as image and video
A multimodal approach is key here, enabling models to work with data in a number of different formats. Technologies such as natural language processing can extract information and feed it to data scientists, enabling them to build and train multimodal AI models to spot relationships between different types of data and share these insights with the business.
A cautious approach
The benefits of GenAI are real and measurable, with less technical users empowered to become more proficient and effective with technology. But business leaders need to use caution in their approach and use models from reputable vendors, within their own security perimeter. By doing so, they can balance risk and reward.
AI is moving fast and it’s an area where organisations cannot afford to stand still. Embracing this technology with caution will enable any business to explore how AI can disrupt their industries, while keeping their business and customers safe.
Related Company Profiles
Snowflake Inc