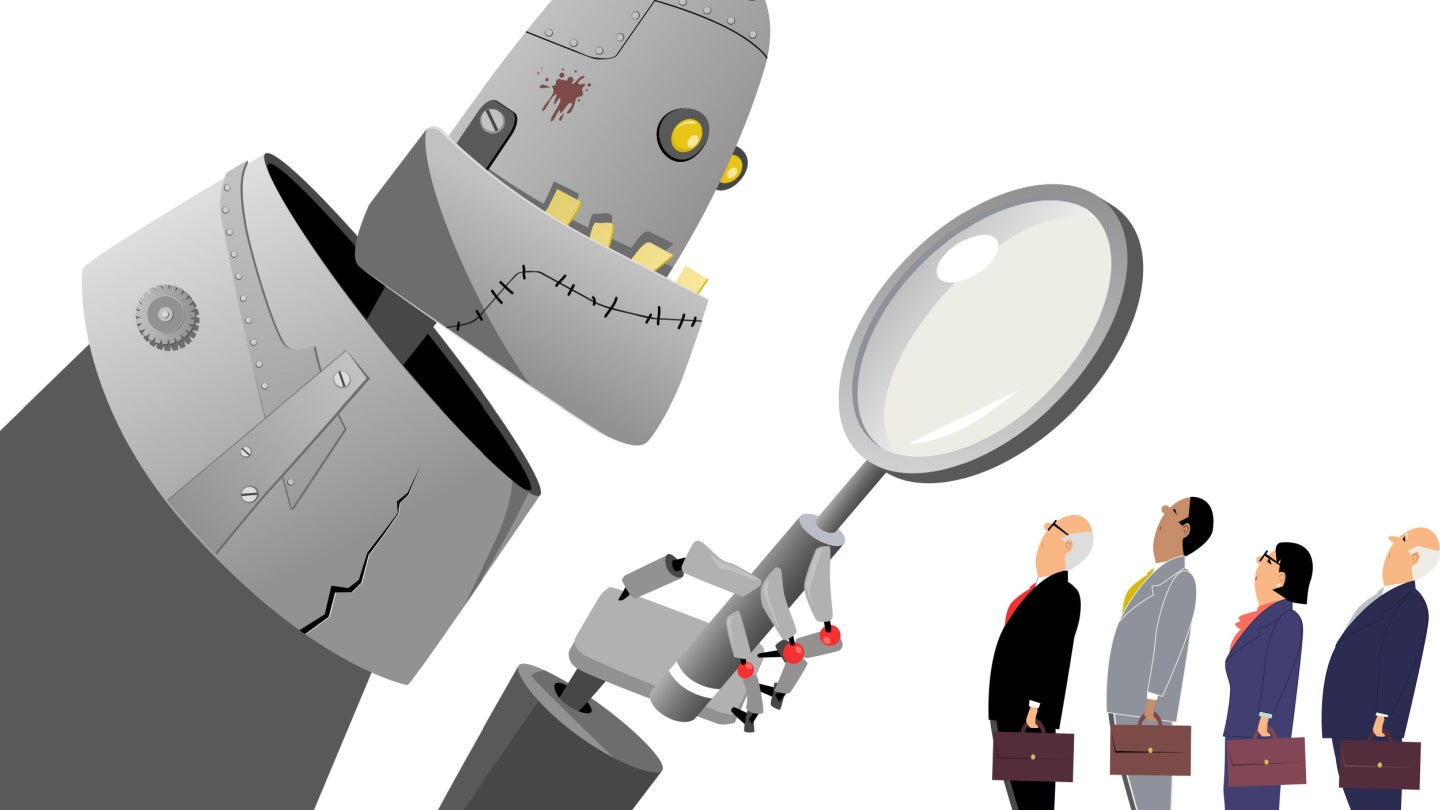
Generative AI has taken off in 2023 with many different industries looking at how they can use it to streamline their operations.
One cross-sector use of generative AI is in AI-enabled recruiting.
This has made it easier for recruiting teams to acquire new talent by quickly sifting through the number of resumes and finding appropriate matches. According to the Institute of Student Employers, 2022 had the highest number of applications per vacancy since it started collecting data in 1999.
In 2022, student employers received an average of 91 applications per graduate vacancy, a 17% increase from the year before. These numbers are often too large for the hiring managers, meaning either an applicant did not get a thorough review, or the hiring process takes too long—the most common hiring complaint.
AI can solve both of these problems as it can automate time-consuming, repetitive tasks while offering personalization and data insights throughout the hiring process.
Which companies are using AI-powered recruiting?
This recruitment technique is already being used by many of the top companies across the globe.
How well do you really know your competitors?
Access the most comprehensive Company Profiles on the market, powered by GlobalData. Save hours of research. Gain competitive edge.
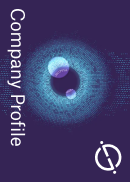
Thank you!
Your download email will arrive shortly
Not ready to buy yet? Download a free sample
We are confident about the unique quality of our Company Profiles. However, we want you to make the most beneficial decision for your business, so we offer a free sample that you can download by submitting the below form
By GlobalDataNotable examples include Amazon, which has developed an automated applicant evaluation system; Hired, which offers an AI platform that matches tech and sales talent with top companies; and Beamery, an AI-powered, talent-management platform that uses AI by aggregating billions of relevant data points to quickly identify and prioritize potential candidates that are likely to thrive at the organization.
Meanwhile, Unilever has stated that it can save hundreds of thousands of pounds by replacing human recruiters with its AI system, reportedly saving 100,000 hours of human recruitment time.
Is there a bias with AI-enabled systems?
To attract applicants, many employers use algorithmic ad platforms and job boards to reach the most ‘relevant’ job seekers. These systems often make highly superficial predictions: they predict not who will be successful in the role, but who is most likely to click that job ad. These predictions can lead to job ads being delivered in a way that reinforces gender and racial stereotypes, even when employers have no such intent.
Examples of this are adverts for supermarket cashiers, which were shown to an audience of 85% women, while jobs for taxi drivers went to an audience of 75% Black candidates.
The act of streamlining candidates can also have bias behind it. In the past, AI models have used a series of ‘knockout questions’ to establish whether candidates are minimally qualified. The problem is that those decisions often reflect the very patterns that many employers are actively trying to change through diversity and inclusion initiatives.
Examples of this can be seen with the video interview process, which amplifies bias toward specific cultural groups. Non-native speakers are often hindered at this stage, with AI labelling the candidate as having poor communication skills. Other examples include bias towards candidates that mention sports more often played by particular sexes and races, or extracurricular activities more often undertaken by the wealthy.
What can be done to prevent this bias?
In the US, there are regulations for companies’ selection procedures. Employers are obligated to inspect their assessment instruments for adverse impacts against demographic subgroups and can be held liable for using procedures that overly favour a certain group of applicants.
However, the responsibility to remove bias lies with the human recruiters. When setting up an AI recruiting model, it is essential to remove any data that introduces inherent bias into the model. Before deploying any predictive tool, recruiters must evaluate how subjective measures of success might adversely shape a tool’s predictions over time. Following that, human beings need to be constantly involved rather than exclusively relying on an automated AI engine, as this will ensure that the process is more balanced.
Beyond simply checking for adverse impacts at the selection phase, employers should monitor the recruitment pipeline from start to finish to detect places where latent bias lurks.
Related Company Profiles
Unilever Plc