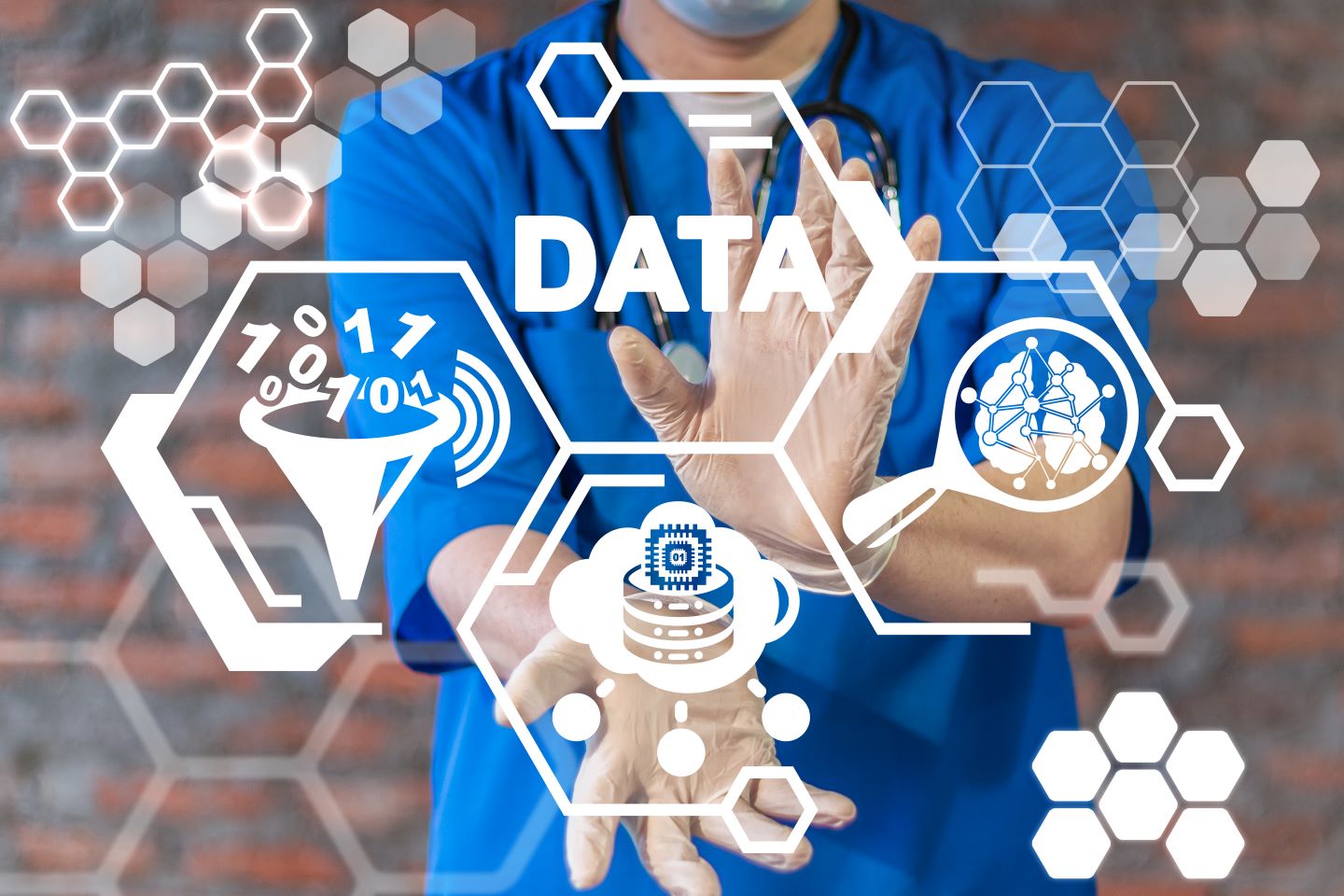
Artificial intelligence (AI) has been a disruptive force, not only in the technology sector but across many verticals for some time. And it has only become more so since the emergence of generative AI in the mainstream, with the release of ChatGPT by OpenAI. Most tech companies are now developing their own generative AI offerings and a vast majority of businesses are exploring the opportunities it offers, as well as the risks it poses. Traditional data analytics vendors have been less vocal, yet disruption has been going on for years before the rise of ChatGPT.
Let’s review how AI has been driving innovation and quietly disrupting the space.
The basics of data analytics
Humans increasingly generate more and more data, largely due to the digitalisation of our society, from databases containing information about citizens in a standardised format to user-created content on social media platforms and sensor data generated by smartphones and industrial machinery. Many industry forecasts expect over 175 zettabytes of data to be generated by 2025. As a result, we are drowning in data, and making sense of so much information is becoming difficult.
The term ‘big data’ has been used for decades to describe extremely large, diverse data sets that, when analysed in aggregate, can reveal patterns, trends, and associations, especially relating to human behaviour and interactions. Big data is characterised by high velocity, volume, value, variety, and veracity—otherwise known as the five V’s of big data.
Data analytics help us go from raw data to useful insights and actionable knowledge. There are effectively four main types of data analytics:
- Descriptive analytics looks at historical data to describe what happened. Techniques used may include clustering, anomaly detection, association rule learning, principal component analysis, and affinity grouping.
- Diagnostic analytics builds on descriptive analytics to answer questions about why things happened. Anomalies and areas of interest are identified and investigated further. Additional related data may be collected, and statistical techniques can be used to explain.
- Predictive analytics classifies future events or estimates unknown outcomes with statistical techniques and machine learning algorithms. Current and historical data are analysed to assess the likelihood of something occurring, including things not on an organisation’s radar.
- Prescriptive analytics is the next logical step after predictive analytics, telling organisations what to do next. By identifying outliers and potential wins and losses, prescriptive analytics recommends actions to improve outcomes. This is often considered the last phase of business analytics.
Not all data analytics are born equal
Because of their heritage and how they use AI, it could be argued that not all data analytics are born equal.
How well do you really know your competitors?
Access the most comprehensive Company Profiles on the market, powered by GlobalData. Save hours of research. Gain competitive edge.
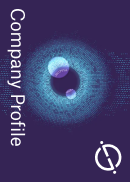
Thank you!
Your download email will arrive shortly
Not ready to buy yet? Download a free sample
We are confident about the unique quality of our Company Profiles. However, we want you to make the most beneficial decision for your business, so we offer a free sample that you can download by submitting the below form
By GlobalDataIn the first wave of AI disruption, machine learning (ML) techniques were used to provide data-driven recommendations by parsing large amounts of data and assessing ‘what if’ scenarios. This innovation enhanced and disrupted the prescriptive analytics field.
Here is where two separate groups of prescriptive analytics vendors can be identified. The first group includes traditional data analytics vendors such as SAS, IBM, MicroStrategy, Oracle, or SAP, which evolved from descriptive analytics roots. Many were founded at the end of the last century, and several as far back as the 1970s, such as SAS and Cognos (now part of IBM).
The second group includes AI-native vendors, such as C3.ai, CognitiveScale, or H2O.ai, which aim to help companies automate operational decision-making using ML. However, this first wave of AI disruption took place in the first decade of the 2000s. For instance, C3.ai was founded in 2009, H2O.ai in 2011, and CognitiveScale in 2013. There were no signs of generative AI at that point, and the Transformer model (the ‘T’ in GPT-3 and ChatGPT) would not be fathered by Alphabet until 2017.
However, with the creation of generative AI, there is arguably a third group also entering the prescriptive analytics space. The large language models (LLMs) of the likes of OpenAI, Alphabet, Meta, Cohere AI, or Anthropic can effectively provide strategic recommendations after ingesting company data as a prompt. While it is still early days, there is a significant opportunity for disruption of the data analytics industry.
Generative AI can be used to either enhance visualisations or to provide recommendations, i.e., prescriptive analytics. Generative AI functionality is being embedded into analytics products to automatically generate preliminary analysis and visualisation from just a textual prompt on a dataset. For instance, ChatGPT has been integrated with Microsoft PowerBI to analyse data sets and automatically generate a brief analysis. Microsoft has launched Microsoft 365 Copilot, embedding ChatGPT into analytics products such as Excel and PowerBI. Arguably, AI has reinvigorated a data analytics market that had long been heading for maturity. Indeed, GlobalData estimates the total data analytics market will be worth $188.8bn in 2027, implying a 13% compound annual growth rate between 2022 and 2027
Related Company Profiles
Alphabet Inc
Microsoft Corp
Meta Platforms Inc
Oracle Corp
SAP SE