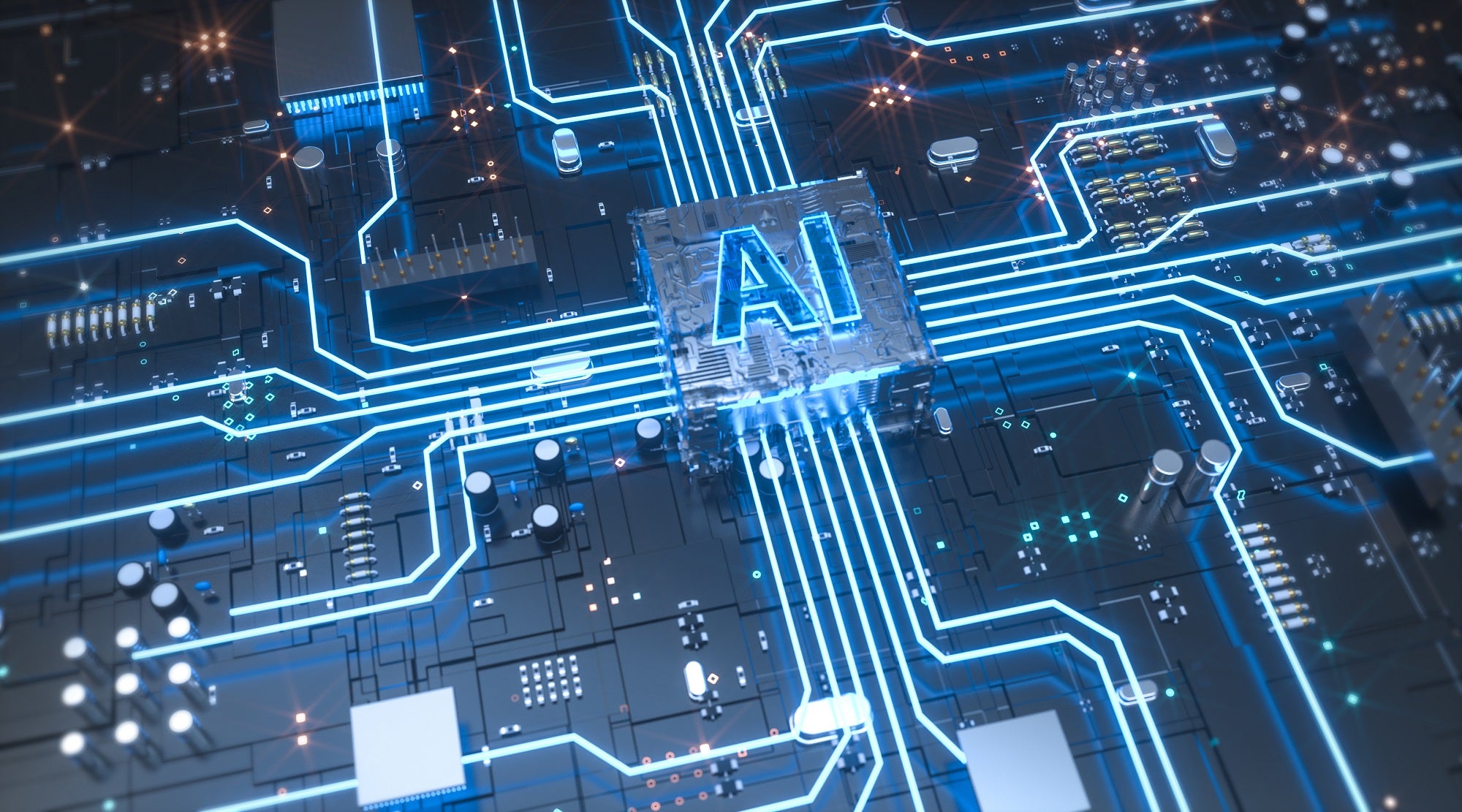
Two new studies have found that when generated data begins to populate the training sets of future AI models, there is a significant degradation in the quality and diversity of the generated output, leading to “model collapse”.
“Model collapse” is a degenerative process whereby models, trained on data polluted by AI-generated data, forget the true underlying data distribution.
One of the studies, “The Curse of Recursion: Training on Generated Data Makes Models Forget”, says that Big Tech companies such as OpenAI and Google benefit from a “first mover advantage” when it comes to training large language models (LLM)s. This is because training of samples from another generative model can induce a “distribution shift”, which causes the model’s predictions to become less accurate over time.
The study, co-authored by researchers at the University of Oxford, the University of Cambridge, Imperial College London and the University of Toronto, emphasises the need to preserve access to the original data source and to continue creating new human-generated data sources.
The authors also suggest the need for “community-wide coordination to ensure that different parties involved in LLM creation and deployment share the information needed to resolve questions of provenance.”
In a blog post discussing the paper, Ross Anderson, professor of security engineering at Cambridge University and the University of Edinburgh, wrote: “Just as we’ve strewn the oceans with plastic trash and filled the atmosphere with carbon dioxide, so we’re about to fill the Internet with blah. This will make it harder to train newer models by scraping the web, giving an advantage to firms which already did that, or which control access to human interfaces at scale. Indeed, we already see AI startups hammering the Internet Archive for training data.”
How well do you really know your competitors?
Access the most comprehensive Company Profiles on the market, powered by GlobalData. Save hours of research. Gain competitive edge.
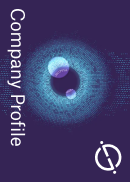
Thank you!
Your download email will arrive shortly
Not ready to buy yet? Download a free sample
We are confident about the unique quality of our Company Profiles. However, we want you to make the most beneficial decision for your business, so we offer a free sample that you can download by submitting the below form
By GlobalData“Large language models”, he writes, “are like fire – a useful tool, but one that pollutes the environment.”
Text to image models are just as susceptible to model collapse
In addition, diffusion models, used by text-to-image companies Midjourney and Stable Diffusion, are just as susceptible to model collapse as LLMs. Another recent study, “Towards Understanding the Interplay of Generative Artificial Intelligence and the Internet”, trained several iterations of diffusion models with a data set composed of elements generated with the previous version of the generative AI model. Working from an original dataset of flowers and birds, the researchers found that there was a progressive degradation in each iteration of the model, first losing details in the first generation, and then ending up in complete noise.
The paper, co-authored by a group of researchers from Spain and Scotland, warns that it will be necessary to accelerate work on the detection of AI-generated content so as to maintain the quality of datasets. “As it stands”, they say, “we are in a race between detection methods and improvements in diffusion models.” Current detection efforts such as watermarking, they say, are not sufficient since it is possible to disable them by using techniques to prevent their readability.