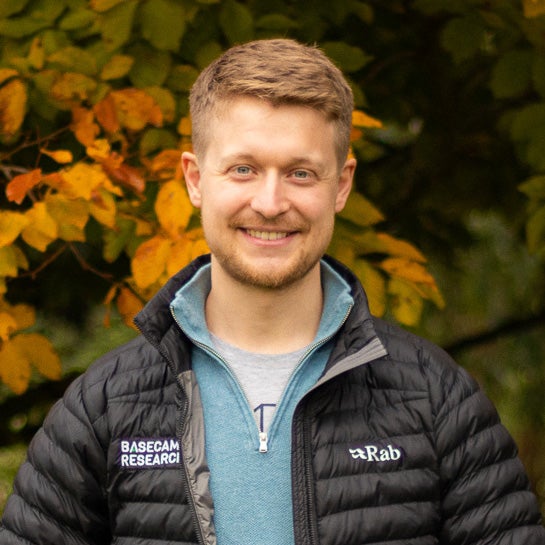
Imagine a company with the ambition to map every single protein the natural world, a foundational dataset that describes all of biology and biodiversity. This is what UK startup Basecamp Research has been doing since its inception in 2019. The company has its research tentacles across the world from Iceland’s ice caps to the African Savanah gathering data about Earth’s molecular diversity to map new and unique protein sequence databases with commercial potential.
The startup’s staff of around 28 comprises ten nationalities, 14 languages, and just under half hold a PhD – with expertise spanning Antarctic ice-divers to machine learning graphic scientists.
Verdict talks to co-founder Glen Gowers about how current AI-based protein design is limited by the datasets currently available, how by mapping nature’s biodiversity and increasing the number of proteins known to science, the company is unlocking the potential for unprecedented discoveries.
What is Basecamp’s origin story?
We started the company with this very big idea of how do we change the way that the biotech industry is developing new products, particularly with AI. We felt very strongly that there’s an elephant in the room within the biotech industry, that we, as an industry, are entirely reliant on public datasets that contain new and novel proteins, and genomes from all over the world. That sounds great but when we dig into what these datasets can do, about half are coming from just 12 species.
These are very well studied species which leads to this really big problem from a data and AI perspective: that we’re working on very small non-diverse datasets. And if we dig in a little bit deeper, these datasets are non contextualised so you have all of these proteins and products that could be being developed, but you don’t know how they relate to each other.
And if we make an analogy with something like ChatGPT, it’s as if we were trying to learn the entirety of the English language, or any language for that matter, by only reading a very, very small subset of a library. What makes ChatGPT powerful is its ability to understand everything, whether on Reddit, Twitter or Wikipedia, for example. It’s very vast and diverse datasets are well contextualised.
How well do you really know your competitors?
Access the most comprehensive Company Profiles on the market, powered by GlobalData. Save hours of research. Gain competitive edge.
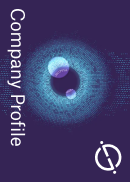
Thank you!
Your download email will arrive shortly
Not ready to buy yet? Download a free sample
We are confident about the unique quality of our Company Profiles. However, we want you to make the most beneficial decision for your business, so we offer a free sample that you can download by submitting the below form
By GlobalDataWhy are publicly held biodiversity datasets a problem for businesses?
The vast majority of foundational layer datasets are almost entirely public datasets that have been accrued by academic work over the last 30-40 years. If you’re searching public data, you can assume your competition is also looking at the same public dataset. There’s an advantage to keeping a dataset like ours private, but very accessible, so that people can come to us with a very low barrier to entry.
How do you use the data you collect?
We build machine learning models on top of this [foundational datasets] and then we partner with companies on delivering solutions for them. So, if they come to us looking for a new enzyme that can synthesize a new drug molecule, we develop that enzyme for them. We can only do that because we have access to this dataset.
How do you build those datasets?
We’re collecting data across 23 different countries, which represents about half of off the planet at the moment, in terms of the diversity of samples. And looking to keep increasing that over time. It’s crazy to us that you can go to a part of the part of the world that is relatively unknown, do a study of what organisms live there, and the majority of it, you have no clue what they are, where the genes come from, what the organisms look like, what species they were, and even probes, by the way, still in a tiny bacteria that we send locally what they do, which speaks to the small Mercer, the size, and lack of diversity of these public datasets, because that’s what you’re comparing against.
We felt there was this big data gap in the biotech sector. And that we had the tools to be able to go and fill that gap by discovering new biology anywhere on the planet. This core dataset that needed to exist was based on our ability to turn biodiversity into something that is currently out there in the world in front of us, into something that’s machine readable. And we can ingest it into machine learning algorithms that require that DNA sequencing gap to be to be at scale around the world.
Your customers include FTSE 100 chemical companies as well as drugs companies. Do your foundational data sets change depending on whether you are working on chemical manufacturing, bioremediation or therapeutics, for example?
No. And that really speaks to why I think the business model exists – it’s a very ‘generalizable’ platform. That hasn’t really been been achieved in biotech before. It’s the same foundational data set. Historically in biotech, to develop a new product, you’d probably need a specific dataset around that type of product, or that type of protein or that market segment. But if you build that dataset correctly, almost like the Wikipedia for biology, and simply build another layer, you don’t need to change the data set, whether you’re going after a molecule for bioremediation, or a molecule that exists as a drug. These are wildly different markets and different types of products, but actually all stemming from the same type of dataset to start with, and that’s really the what opens up a new business model that we’re pursuing here at a platform level.
Are there any use cases that might surprise people?
There are applications we don’t even know about yet. A chemical manufacturing plant or a therapeutic drug discovery program is something that doesn’t really appear on your shelf in Tescos. But we are seeing a move towards consumer based synthetic biology or biotech products. For example, there’s one company we know selling an engineered plant, and people will be able to buy and have that in their homes to filter air in a new way. All these products rely on new new proteins, new genomes and new organisms is being discovered and then being made accessible and commercializable to them.
What would you say to potential clients, who didn’t necessarily want to the financial commitment of this technology at the moment – can they experiment nevertheless?
We run high level sort of scoping exercises with various different clients, everything from small startups to huge corporates where we want to make sure that we are a innovation partner for these companies, right. And so many will turn into paying customers that we work on specific products with. But we also want to stretch the platform as far as possible. So we have lots of companies coming to us saying they are thinking about a new product and asking: What does nature have? What can I learn from that? What is your dataset describing around this space? We can do that quickly without any money changing hands to almost ideate with our partners. Many turn into actual projects and products. But many of them may just stay as ideas. And that’s, great. That’s our platform.