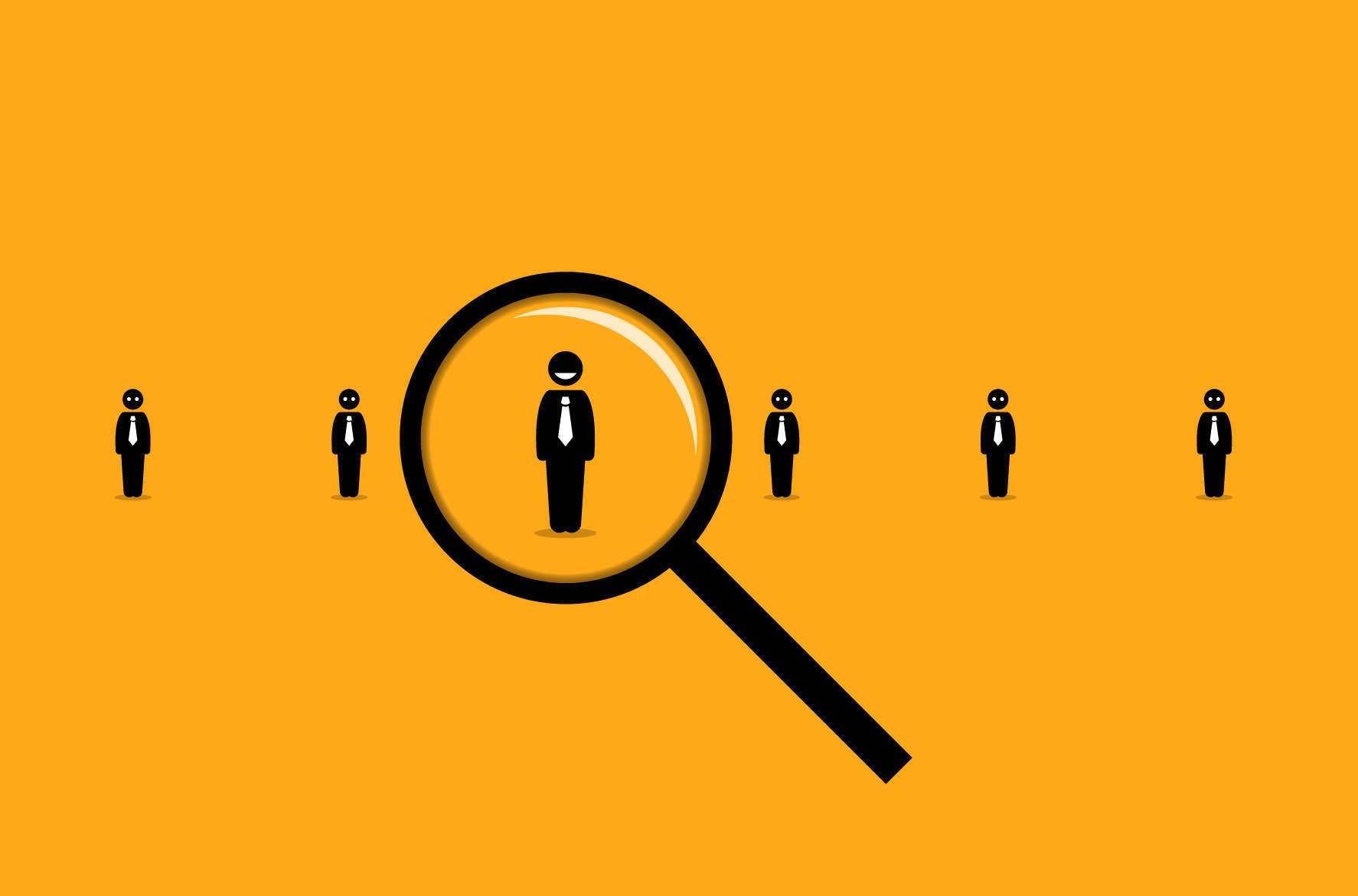
Amazon’s decision to scrap its AI recruiting tool after discovering that it was biased against women has shed light on the problems with acquiring effective training data, and why it’s so important to have good quality datasets.
Designed to sort through the tech giant’s unwieldy number of applications to find those best suited for given positions, the AI recruiting tool was considered a “holy grail”.
However, in 2015, although revealed by Reuters today, Amazon discovered it had a problem: the tool was sexist, assigning a negative score terms that included the word “women’s” – including attendance in women’s clubs and even all-women colleges.
The reason behind this was simple: Amazon had, in good faith, trained the tool on past resumes, with it identifying patterns that signalled a high-quality candidate. But as most of these were from men due to the male-dominated nature of the tech industry, it resulted in the AI being biased against women.
The project was, as an Amazon spokesperson confirmed, “never used by Amazon recruiters to evaluate candidates,” and was only ever used in trials. It was ultimately abandoned for a number of reasons, including the fact that it failed to product strong candidates.
However, the issues with bias do shed light on the wider problems surrounding training data.
How well do you really know your competitors?
Access the most comprehensive Company Profiles on the market, powered by GlobalData. Save hours of research. Gain competitive edge.
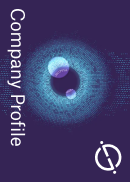
Thank you!
Your download email will arrive shortly
Not ready to buy yet? Download a free sample
We are confident about the unique quality of our Company Profiles. However, we want you to make the most beneficial decision for your business, so we offer a free sample that you can download by submitting the below form
By GlobalDataWhen an AI recruiting tool becomes sexist
When artificial intelligence tools are trained, they are fed large batches of data that they use to identify patterns and build up a picture of what their right method of action should be – whether it is sorting resumes into ‘good’ and ‘bad’, identifying cybersecurity threats or answering a customer query.
Training is generally something that is done repeatedly over time, with improvements made along the way, but there are always two vital factors: the size of the dataset and the quality of the dataset. And for resumes this means a dataset that effectively covers all potential applicants, not just a subset of them.
“Successful AI requires quality, diverse training data, because the ‘garbage in, garbage out’ concept is critical when building algorithms,” explained Dr Steve Arlington, President of The Pistoia Alliance.
“You can’t expect high quality outcomes if low-quality data is being fed to AI. Modern machine learning algorithms can deal with dirty or incomplete data to a certain extent, but even so the importance of quality training data cannot be overestimated,” added João Graça, CTO of Unbabel.
AI training data and the diversity challenge
Unfortunately, getting large, high-quality and diverse datasets is often a significant challenge. In fact, access to robust, diverse datasets could be argued to be the technology industry’s biggest unmet need.
“Good data is representative, diverse and clean. That is, it relates to your industry or area of work, covers everything you want to achieve, and doesn’t contain any bias,” said Graça.
“In many cases, businesses fall down because they don’t have provide their AI enough examples of ‘desirable outcomes’. If you are building a recruitment system which automatically predicts candidate suitability, representative examples for both men and women should have been a no-brainer.”
For niche applications, such as a recruiting tool for a specific company or a healthcare tool for an unusual illness, a robust, genuinely diverse dataset of adequate size quite often simply doesn’t exist.
“Amazon’s issue is with recruitment, but the challenge is highly relevant to other industries, too,” said Arlington. “Take the life sciences and healthcare sector for example – when AI is making decisions about people’s health the need for a correct, impartial response is paramount.
“In clinical trials, there are worries that recruitment is not representative of demographics. This is a problem given that age, race, sex, and more, play a vital role in a person’s response to a drug.
“One report found that although since the 90s, the number of countries submitting clinical trial data to the FDA has almost doubled, the equivalent increase hasn’t been seen in the diversity of the clinical trial population – in 1997, 92% of participants were white, the figure in 2014 was 86%. Additionally, adult males also dominate the clinical trial population, representing about two thirds.”
For every industry, then, there is the need to build better and more diverse datasets.
“The diversity of data in all industries must be improved to ensure we are training and building AI algorithms that will provide the best recommendations for all groups,” he added.
Legislative barriers to training data
However, it is not as simple as just building more datasets. Despite the vast amounts of data generated each day, much of which would be invaluable to the right industry, access to data is often extremely hard to obtain due to the legal protections in place.
“New legislation, like GDPR, is making data sharing near impossible and therefore limits startups’ access to the fuel needed to train their algorithms and get products customer-ready,” said Peony Li, head of investment at Founders Factory.
“New privacy and data protection laws closely monitor what data is captured and shared, and this is causing a roadblock to new technology’s use of big data.”
For some, the solution is to anonymise data, however Li argued that this often damages its effectiveness for training AI.
“Anonymisation doesn’t just reduce the quality of data (which is especially acute for sparse datasets), it also compromises the accuracy of insights that can be inferred. That can lead to the targeting the wrong group of customers for a big marketing campaign or a wrong product launch, which can cost up to millions.”
Is synthetic data the answer?
There may be a solution to the problem, however, in the form of synthetic data, which is based on real-world data but provides a more reliable, scalable alternative to anonymised data.
“Synthetic data mimics the statistical properties of original data while keeping it secure,” explained Li.
“Insights obtained from the synthetic data will be similar to insights from original data, and the synthetic aspect frees up the data to be shared openly without disclosing individual-level sensitive information.”
Significantly this also allows the size of datasets to be increased without needing to find new sources. And given how important large datasets are to good AI training, this could be significant.
“Synthetic data can solve, to a certain extent, the stalemate in scaling datasets,” she added.
“As a startup there can be some difficulties in getting data needed to train their product unless they have pilot customers willing to give up valuable data for an external untrained ML algorithm. With synthetic data, AI startups can now turbocharge their algorithm much earlier.”
AI recruiting tools in the spotlight
Even if datasets can improve AI recruiting tools training, the practice of using such technologies now sits in the spotlight. But despite the failure of Amazon’s efforts, the technology still has significant value for the field – as long as it’s used with caution.
“In many ways, technology has made the job-seeking and hiring process easier than ever before; however, with these advancements have also come a set of added challenges for both candidates and the organisations who want to hire them,” said Amanda Augustine, career advice expert for TopCV.
“As the use of robotics – including AI technology – during the recruitment process continues to increase, it’s imperative that employers and the makers of such technology continually question the science behind the tools – and scrap any recruiting tool which shows a bias of any kind.”